Technological advances such as machine learning and big data models can improve our understanding of current economic conditions. Nowcasting models bridge the data gap between statistical releases, helping to identify trends in real time and allowing more accurate and timely policy measures.
To understand the behaviour of the economy and to predict future trends in key indicators, such as GDP, economists use macroeconomic modelling and forecasting, which involves building and analysing economic models (mathematical/statistical representations of the economy).
These tools help policy-makers to make informed decisions by simulating the effects of various scenarios or policies. When we talk about macroeconomic modelling and forecasting, it is important to remember that there are different models used for different objectives. There is not a single ‘best’ model, and usually, the best strategy is a multi-model approach.
Indeed, the global financial crisis of 2007-09 highlighted the limitations of traditional economic modelling. It opened the door to the introduction of financial models and the inclusion of the financial sector, frictions and variables in macroeconomic modelling.
Over the past decade, the emergence of data-rich environments has been rapid and widespread. This process has included the use of big text and data analysis in economic modelling, with two important revolutions: Bayesian econometrics; and data and machine learning.
Bayesian approaches can be used to estimate the probability of an event, incorporating prior knowledge and/or historical data. The analyses balance flexibility and interpretability, sitting between causal models (which prioritise understanding the underlying mechanisms that are driving economic relationships) and more data-driven approaches (see Figure 1).
Large language models and machine learning provide strong forecasting power, excelling in pattern recognition and prediction. But they do not provide clear causal explanations. Both Bayesian methods and machine learning complement traditional causal approaches by enhancing interpretability and predictive accuracy in different contexts.
Figure 1: Subjective evaluation of models
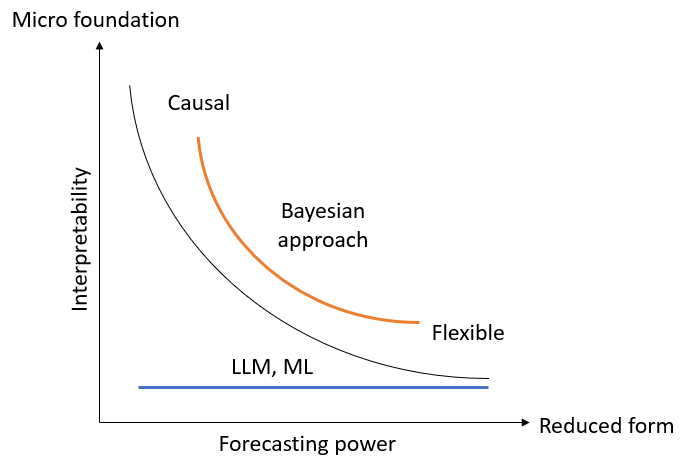
Source: Luca Onorante, Workshop on Macroeconomic Analysis and Forecasting for Policy and Practice, University of Strathclyde, November 2024
Note: LLM = large language model; ML = machine learning
Forecasting requires more than just modelling: it is a combination of technical, economic knowledge and judgement. But new modelling techniques can help. Machine learning techniques, artificial intelligence (AI) and big data are especially useful for forecasting in the short term or non-normal times, such as when there is a recession or a big ‘exogenous shock’ (an unexpected economic disruption) like the Covid-19 pandemic. In these periods, policy-makers are looking for approaches that allow real-time monitoring of the state of the economy.
What is nowcasting?
Nowcasting refers to the use of recently published data to update key economic indicators that are published with a lag – such as real GDP – to predict economic trends as they happen. Nowcasting has been used in meteorology for a long time, defined as the detailed analysis and description of the current weather and the forecasting ahead for a period of up to six hours.
It has become relevant in economics as key statistics on the present state of the economy are usually available with a significant delay, which can create uncertainty. The idea behind nowcasting is to use information that is published early and at a higher frequency to obtain an early estimate before the official numbers are released.
For example, if the target variable is GDP, then information about expenditure or consumption can be used, as this is normally released monthly (compared with the quarterly release of official GDP statistics). Other information, such as surveys or financial variables, can be another source of timely and frequent data. The idea is that information from higher frequency variables together with surveys provide an early indication of the present state of economic activity.
How does it help macro-forecasting?
Nowcasting can be useful to produce more timely and appropriate policy measures during crisis periods, such as the global financial crisis or the pandemic. In addition, it can be used to study of the role of financial variables in macroeconomic forecasting, as it considers the timeliness of financial information.
Another advantage of nowcasting is that it can be incorporated in structural environments. One example would be in ‘dynamic stochastic general equilibrium’ (DSGE) models, which have been developed and used widely for macroeconomic forecasting, especially at central banks.
Doing so allows the computation of real-time estimates of variables in the model that are not directly observable, such as the output gap or the natural rate of unemployment. The combination of imputing these data through the structural model can make it possible to identify important shocks to the economy in real time.
How is nowcasting used in the UK economy?
Nowcasting is used by government, which is typically interested in an understanding of growth across the whole economy (aggregate growth). A recent workshop heard from HM Treasury and the Scottish Fiscal Commission about their use of nowcasting methods, and from researchers at the University of Strathclyde about how they are developing their existing work for applications in Scotland.
The Scottish Fiscal Commission, as Scotland’s fiscal ‘watchdog’, has a need to nowcast Scottish GDP growth for the current financial year.
Sub-national nowcasting presents several challenges, principally around data. These include longer release delays, not all national-level data being available at a sub-national level or the data having a shorter historical coverage.
This leads to ‘missing’ data at the start and end of the dataset. An additional practical challenge is that the available data differ in their frequency (some are annual, others quarterly or monthly). This demands the use of ‘mixed-frequency’ methods.
Working with colleagues at the University of Strathclyde, the Scottish Fiscal Commission is exploring whether models developed by these researchers may be able to be used in its work. The model being adapted to produce nowcasts of Scotland's quarterly GDP growth rates is a mixed-frequency vector autoregression (VAR) model, which combines low-frequency (annual) data and high-frequency (quarterly or monthly) data.
The model uses as predictors a range of macroeconomic variables including the UK growth rate, inflation, the interest rate, the exchange rate and the oil price, alongside a series of regional factor variables based on a large dataset of regional indicators. It is hoped that this approach will help to capture dynamics within Scotland’s economy more effectively.
HM Treasury also uses nowcasting frameworks, specifically the first estimate of GDP focusing on expenditure, income and output. Output variables are essential for the first estimate, with data being reported quarterly in the national accounts.
To predict GDP one quarter ahead, the framework uses an industry nowcast that relies on sector and industry-level data, which together make up the output measure of GDP. This model also incorporates top-down judgments to adjust for extraordinary events – such as unexpected economic disruptions – that may not be reflected in the regular data sources. This method ensures that the model remains relevant and responsive to real-world disruptions.
A key feature of this framework is the use of the mixed data sampling (MIDAS) model, which integrates data at mixed frequencies (both quarterly and monthly GDP). By modelling each indicator separately, the MIDAS approach combines these individual predictions into a single nowcast, using a weighted average approach. This is then used in the direct estimation of quarterly GDP.
Beyond an assessment of aggregate movements in the economy, there is growing interest in distributional outcomes (how people at different points in the income distribution might be affected in different ways). The workshop heard about one current project working to do this.
Its approach has two aims: first, to improve the timeliness of our understanding of the income distribution in the UK; and second, to provide a model that enables us to explore its resilience to economic shocks (De Polis et al, 2024). To date, the team has achieved the first aim for this project.
Until recently, understanding how economic shocks affected different segments of the population was a focus in microeconomics. Recently, macroeconomics has begun to link models of the macroeconomy to microdata to enable the analysis of macroeconomic shocks on distributional outcomes (that is, where outcomes like income, productivity or turnover differ across individuals/firms). The mixed-frequency functional VAR method being developed aims to shed new light on how economic shocks affect individuals at different points of the income distribution.
A significant challenge for policy-makers is the time lag in microdata availability. Microdata that can shed light on population characteristics take longer to produce and release than aggregate economic survey metrics. The survey used by the research team is released with an average delay of a year and a half.
This delay can hinder detection or understanding of how economic shocks affect the income distribution in real time, delaying necessary policy responses. To address this, the study develops a state-space model that links changes across the whole economy (aggregate economic changes) with effects at different income levels (distributional dynamics) at business cycle frequencies.
Using income survey data from the Office for National Statistics (ONS) Living Cost and Food Survey (and its predecessor surveys, which date back to the 1970s), the model provides a timely nowcast of the underlying micro-distribution, enabling real-time predictions of changes in the income distribution over time.
As more data are incorporated, prediction errors are reduced, providing policy-makers with more accurate and timely insights to plan and implement responses to economic changes more effectively. The next stage in the research project is to use this model to understand how economic shocks affect individuals at different points in the income distribution.
Conclusion
Incorporating new data-driven techniques – such as nowcasting, machine learning and AI – into macroeconomic forecasting can significantly improve the accuracy and timeliness of economic analysis.
By combining traditional economic models with these innovative approaches, policy-makers can gain deeper insights into economic conditions in real time, improving their ability to respond effectively to crises and unexpected shocks.
While challenges such as data limitations, methodological integration and ensuring interpretability remain, the evolution of macroeconomic forecasting will rely on a multi-modelling approach, blending the strengths of different methodologies to achieve a more comprehensive and resilient economic outlook.
Where can I find out more?
- Developing nowcast methodologies for public service productivity in the UK: Overview from the ONS.
- Gauging the globe: the Bank’s approach to nowcasting world GDP: Bulletin from the Bank of England.
- Regional nowcasting: Estimates available via the Economic Statistics Centre of Excellence (ESCoE).
- Forecasting and nowcasting macroeconomic variables: A methodological overview: Working paper by Jennifer Castle, David Hendry and Oleg Kitov.
- Real-time probabilistic nowcasts of UK quarterly GDP growth using a mixed-frequency bottom-up approach: Article from the National Institute of Economic and Social Research (NIESR).